The Role of AI in Drug Discovery: Accelerating the Pipeline
13 Feb, 20255 minsArtificial intelligence (AI) is slowly finding its way into a widening array of fields. Its ...
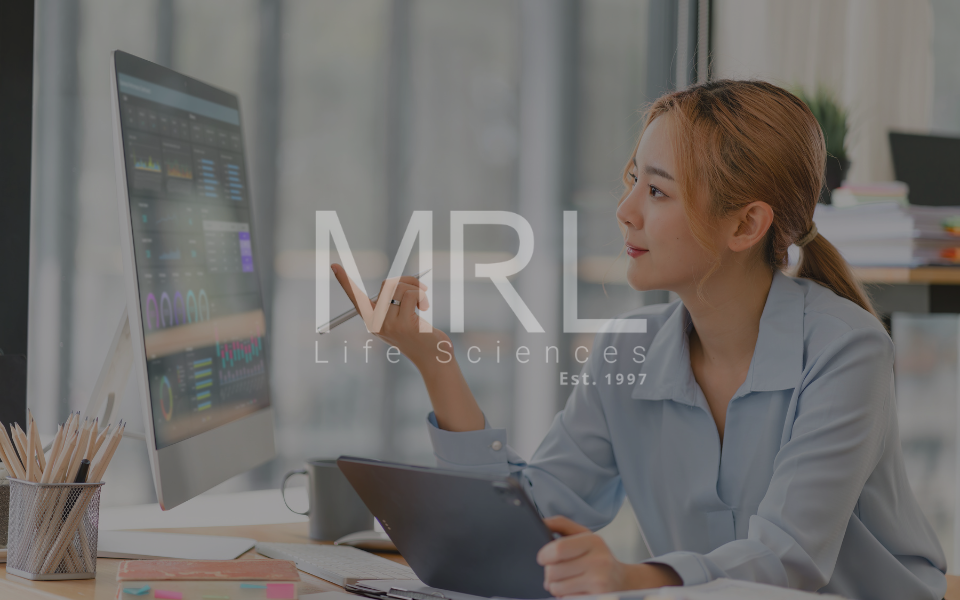
Artificial intelligence (AI) is slowly finding its way into a widening array of fields. Its accuracy and ability to work at volume and speed are proving invaluable across sectors. So, it’s little surprise that AI is now playing an increasingly pivotal role in accelerating the drug discovery pipeline. What has traditionally been a lengthy and costly process is undergoing dramatic efficiencies, with timeframes falling and the potential for success increasing.
How AI is Accelerating the Drug Discovery Pipeline
Target identification
Perhaps the most widely used feature of AI is data processing. Because it can sift through vast amounts of biological and chemical data, it can be used to rapidly identify promising targets for new drugs. Using machine learning (ML) to detect patterns it may take human researchers years to uncover, AI expedites the entire target identification and validation process.
Reaction prediction and drug design
Thanks, again, to its ability to process enormous amounts of data, AI models can be created to predict how drugs may impact the conditions and the people they are intended for. By modelling how proteins or other molecular targets might interact with drugs, scientists can use AI to validate the drugs before proceeding with development. This is traditionally one of the most labour-intensive aspects of drug discovery. Deep learning algorithms can reduce that part of the drug discovery lifecycle dramatically.
Predicting toxicity and side effects
It is estimated that 90% of clinical trials fail. Which is one of the reasons why the process is so expensive. AI is helping to address that by predicting the safety profile of drug candidates before trial. Machine learning models can detect patterns of toxicity that may not be immediately obvious, while AI can analyse historical data on known drugs and their side effects. Drastically reducing the risk of clinical trial failures and improving overall drug safety.
Biomarker discovery
Biomarkers are crucial for developing personalised medicine—something that has received a lot of attention recently. With AI being able to analyse patient data, including genomics, proteomics, and clinical outcomes, to identify potential biomarkers, the discovery process time is reduced. And designing targeted therapies becomes easier.
Clinical trial improvement
AI holds the potential to improve a couple of elements of clinical trials. Its ability to research and refine allows for better patient identification and recruitment in the first instance. While its proficiency at real-time data monitoring and analysis means that it can flag any potential issues early, enabling researchers to adapt the study design if necessary.
Repurposing existing drugs
Multiple drugs have been found to have a range of applications. Whether it’s epilepsy drugs for pain relief, or hair loss drugs for prostate health. By analysing all available data, including clinical trial results, scientific literature, and genetic information, AI can rapidly facilitate the repurposing of drugs.
Predicting drug interactions
In the same way, AI can be used to predict interactions between drugs and how they may effect patients. By analysing large datasets from pharmacological and clinical studies, AI algorithms can assess how drugs interact at the molecular level, providing insights that would be time-consuming to obtain through experimental methods alone.
Accelerating time to market
The combination of all of these benefits can work to reduce the overall time it takes to bring a drug to market. In some cases, this may also reduce the costs involved in this process and the end cost to patients.
The integration of AI into drug discovery is still a relatively new process. But it holds enormous potential. The automation of data analysis, improved target identification, the reduction of side effects, and the optimisation of clinical trials hold the key to transforming the pharmaceutical industry.
Find out more about becoming part of the exciting future of the pharmaceutical industry with MRL’s life sciences vacancies.